Dr. J. Michael Herrmann- Self-organised Criticality in Neural Systems and Autonomous Robots
Title: Self-organised Criticality in Neural Systems and Autonomous Robots
Speaker: Dr. J. Michael Herrmann, The University of Edinburgh, U.K.
Chair: Dr.Shan Yu, Brainnetome Center, CASIA
Time: 09:30-10:30 August 18, 2016
Venue: 714 meeting room, 7th floor of the Intelligence Building
[Abstract]
The phenomenon of self-organised criticality characterises the dynamics of systems as diverse as sandpiles, earthquakes, ecosystems, animal swarms, financial markets and the brain. It is particularly interesting in neural networks where criticality can be shown to lead to optimal transmission and storage of information. Moreover, critical neural networks can improve sensing and control when used in autonomous robots. Operation at or near a critical point enables a robot to generate a sequence of optimal training data for learning a model of the environment. The dynamics of this control system is analysed in theory, in robotic experiments and discussed as an abstract model of brain function. Following the idea of guided self-organisation, we show how pattern formation in critical systems can improve the learning abilities when an external evaluative signal is available.
[Biography]
Dr. Michael Herrmann received a Diploma (1988) from the University of Leipzig and a Doctorate for the same institution (1993). He was working as a research assistant from 1992 and as a postdoc in Denmark and Japan. His PhD focused on mathematical aspects of artificial neural networks. In 2008, after a temporary position as an assistant professor at the University of Goettingen, he was appointed to the post of Lecturer in Robotics at the School of Informatics at the University of Edinburgh.
Dr. Herrmann is currently principal supervisor for five PhD students at the University of Edinburgh and has acted as principal or co-supervisor for ten successful PhD students mainly at the University of Goettingen. He has also supervised several MSc students. In recent years, he has taught courses on reinforcement learning for MSc and final year undergraduates, as well as on natural computing for third year undergraduates and is co-teaching an introductory course on vision and robotics also for third year undergraduates.
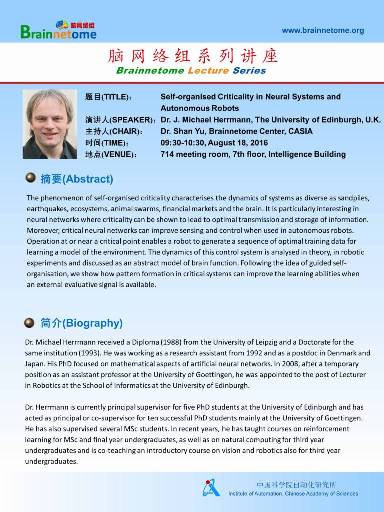