Dr. Zhang's Lecture -- Causal discovery in light of different types of "independence"
Title: Causal discovery in light of different types of "independence".
Speaker: Dr. Kun Zhang
Chair: Prof. Tianzi Jiang
9:00-10:00 June. 24, 2014
Venue: The first meeting room, 3rd floor of the Intelligence Building
If you want to attend it, please contact to Dr. Yong Liu by yliu@nlpr.ia.ac.cn
[Abstract]
This talk is concerned with three types of "independence" that makes it possible to discover causal information from purely observational data. The traditional constraint-based causal discovery approaches rely on conditional independence relationships and produce an equivalence class of the underlying causal structure. Recently a class of causal discovery methods based on functional causal models has been proposed, which, under certain conditions, is able to fully identify the causal structure. Generally speaking, those methods make use of additional properties of a causal system other than conditional independence relationships. Specifically, two types of "independence" in the functional causal models help tell cause from effect: they are statistical independence between the cause and noise (independent noise) and independence between the distribution of the cause and the transformation from the cause to the effect (independent mechanism). In this talk I will show how the above three types of "independence" -- namely, conditional independence, independent noise, and independent mechanism, enable causal discovery, and further discuss connections between them.
[Biography]
Dr. Kun Zhang is currently a senior research scientist at Max Planck Institute for Intelligent Systems, Germany. His main research interests include causal discovery, machine learning, neuroscience, and computational finance. He, together with his colleagues, has made a series of important contributions towards solving some long-standing problems in causality, such as how to distinguish cause from effect in the two-variable case and nonparametric conditional independence test. He received the best benchmark award of the second causality challenge and co-authored the best student paper at UAI 2010, and has been the (co-)organizer of a series of workshops in causality.
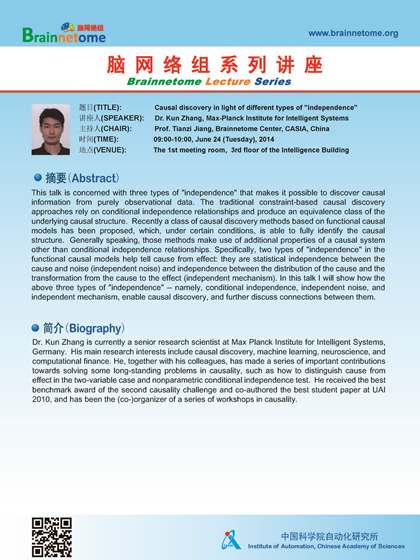