Prof. Weng's Lecture -- Brain Does Not Use a Deep Cascade of Areas: The Deep Learning Architectures Are Shortsighted
Title: Brain Does Not Use a Deep Cascade of Areas: The Deep Learning Architectures Are Shortsighted
Speaker: Prof. Juyang Weng, Michigan State University, USA
Chair: Prof. Tianzi Jiang
Time: 09:00-10:00 Dec. 30, 2013
Venue: The first meeting room, 3rd floor of the Intelligence Building
If you want to attend it, please contact to Dr. Yong Liu
[Abstract]
There have been many deep learning networks, e.g., Cresceptron by Weng et al. 1992, Multilayer Convolution Network by LeCun et al.1998 and Deep Autoencoder Network by Hinton et al. 2006. They are characterized by a deep cascade of processing areas.
In this talk, I explain that the brain architecture is not a cascade of proceeding areas, but rather a network that involves both “shallow” and “deep” learning pathways. Further, the brain wires “shallow” pathways before wire “deep” pathways. Based on our brain-inspired DN (Developmental Network), I explain that the DN takes the best of the two schools of Artificial Intelligence (AI) --- the symbolic AI school and connectionist AI school. I present why the framework of finite automaton is general-purpose, going behind the Turing Machine framework, because it not only copes with abstract rules (e.g., syntax) but also meanings (e.g., semantics). Furthermore, neural modulation, such as serotonin, dopamine, acetylcholine, and norepinephrine systems, is critical for motivation (including emotion) integrated into DN in a mathematically well-defined way. I will also present some recent experimental results for vision and language learning.
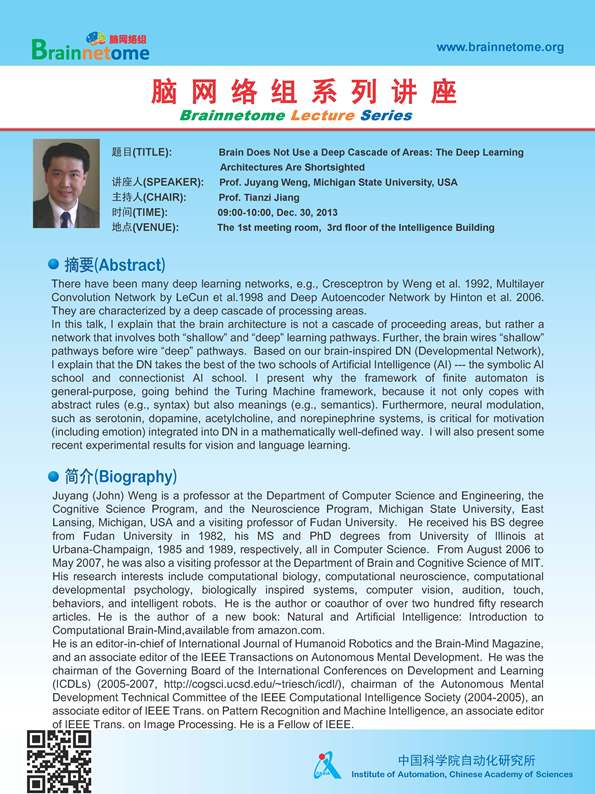